F-distribution
TBA How much finished
10%
Background
A number of vignettes used to be part of the RJafroc
package. These have been moved here.
Introduction
Since it plays an important role in sample size estimation, it is helpful to examine the behavior of the F-distribution. In the following ndf
= numerator degrees of freedom, ddf
= denominator degrees of freedom and ncp
= non-centrality parameter (i.e., the \(\Delta\) appearing in Eqn. (11.6) of (Dev P. Chakraborty 2017)).
The use of three R
functions is demonstrated.
qf(p,ndf,ddf)
is the quantile function of the F-distribution for specified values of p
, ndf
and ddf
, i.e., the value x
such that fraction p
of the area under the F-distribution lies to the right of x
. Since ncp
is not included as a parameter, the default value, i.e., zero, is used. This is called the central F-distribution.
df(x,ndf,ddf,ncp)
is the probability density function (pdf) of the F-distribution, as a function of x
, for specified values of ndf
, ddf
and ncp
.
pf(x,ndf,ddf,ncp)
is the probability (or cumulative) distribution function of the F-distribution for specified values of ndf
, ddf
and ncp
.
Effect of ncp
for ndf
= 2 and ddf
= 10
- Four values of
ncp
are considered (0, 2, 5, 10) for ddf
= 10.
fCrit
is the critical value of the F distribution, i.e., that value such that fraction \(\alpha\) of the area is to the right of the critical value, i.e., fCrit
is identical in statistical notation to \({{F}_{1-\alpha ,ndf,ddf}}\).
ndf <- 2;ddf <- 10;ncp <- c(0,2,5,10)
alpha <- 0.05
fCrit <- qf(1-alpha, ndf,ddf)
x <- seq(1, 20, 0.1)
myLabel <- c("A", "B", "C", "D")
myLabelIndx <- 1
pFgtFCrit <- NULL
for (i in 1:length(ncp))
{
y <- df(x,ndf,ddf,ncp=ncp[i])
pFgtFCrit <- c(pFgtFCrit, 1-pf(fCrit, ndf, ddf, ncp = ncp[i]))
}
for (i in 1:length(ncp))
{
y <- df(x,ndf,ddf,ncp=ncp[i])
curveData <- data.frame(x = x, pdf = y)
curvePlot <- ggplot(data = curveData, mapping = aes(x = x, y = pdf)) +
geom_line() +
ggtitle(myLabel[myLabelIndx]);myLabelIndx <- myLabelIndx + 1
print(curvePlot)
}
fCrit_2_10 <- fCrit # convention fCrit_ndf_ddf
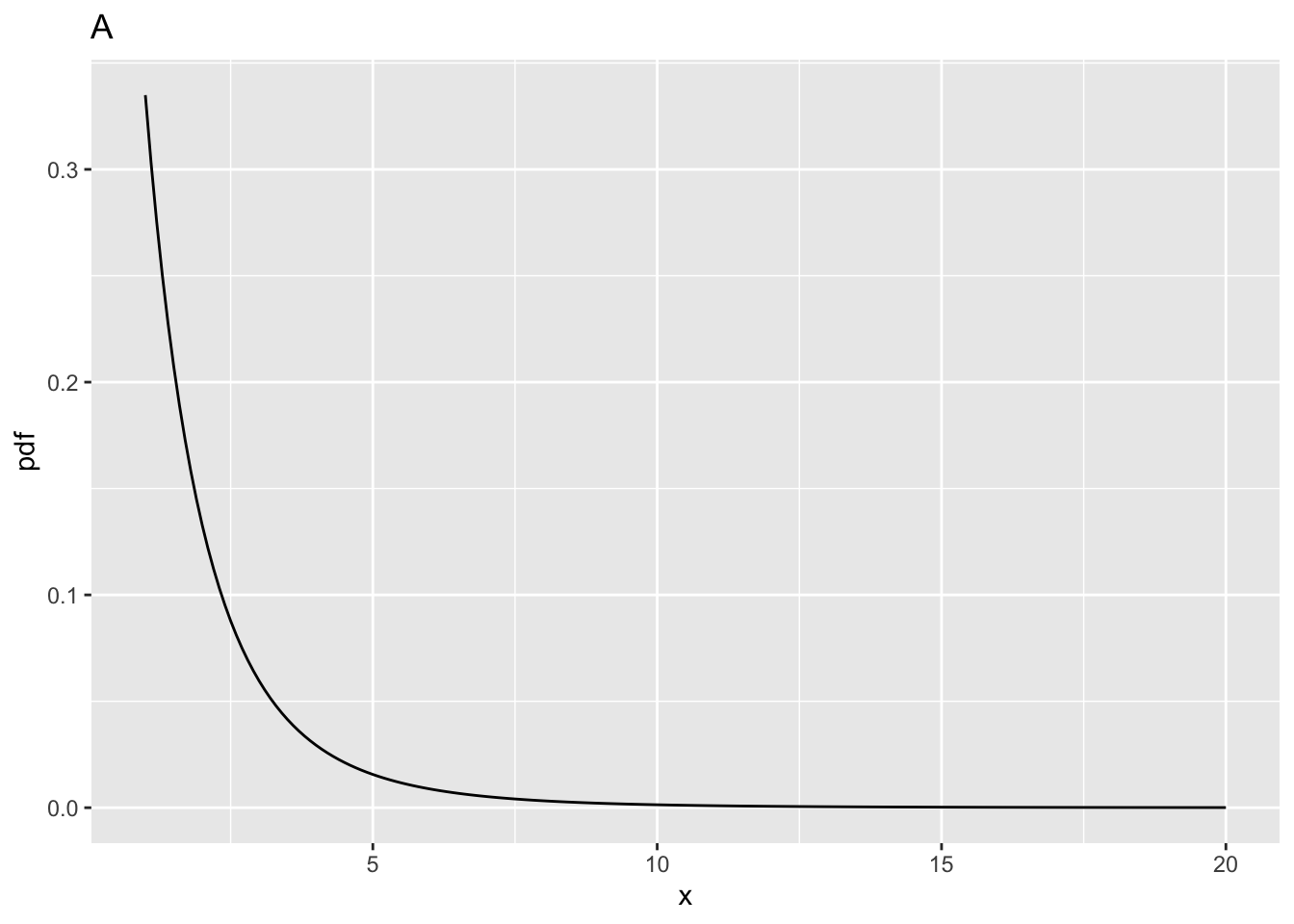
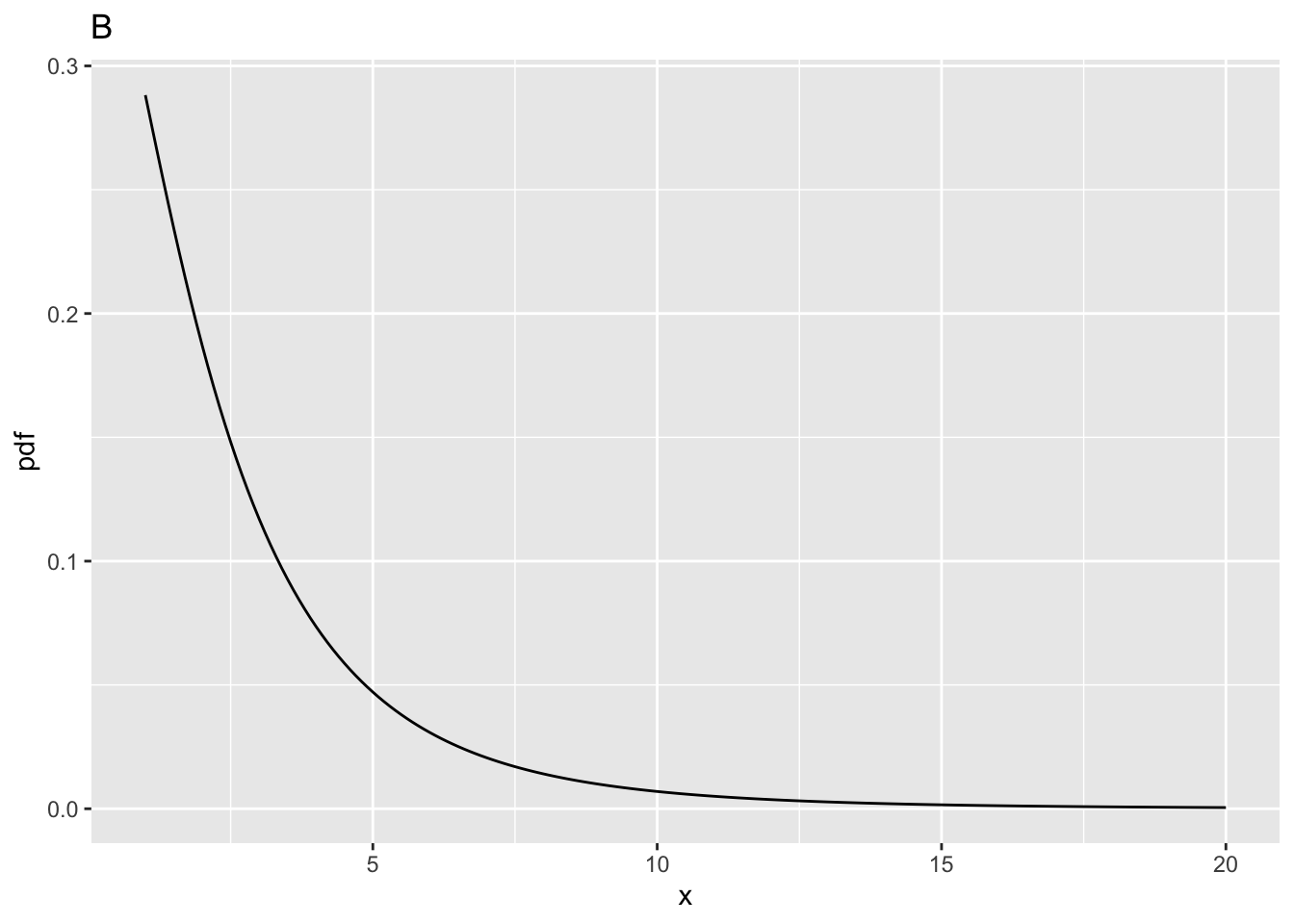
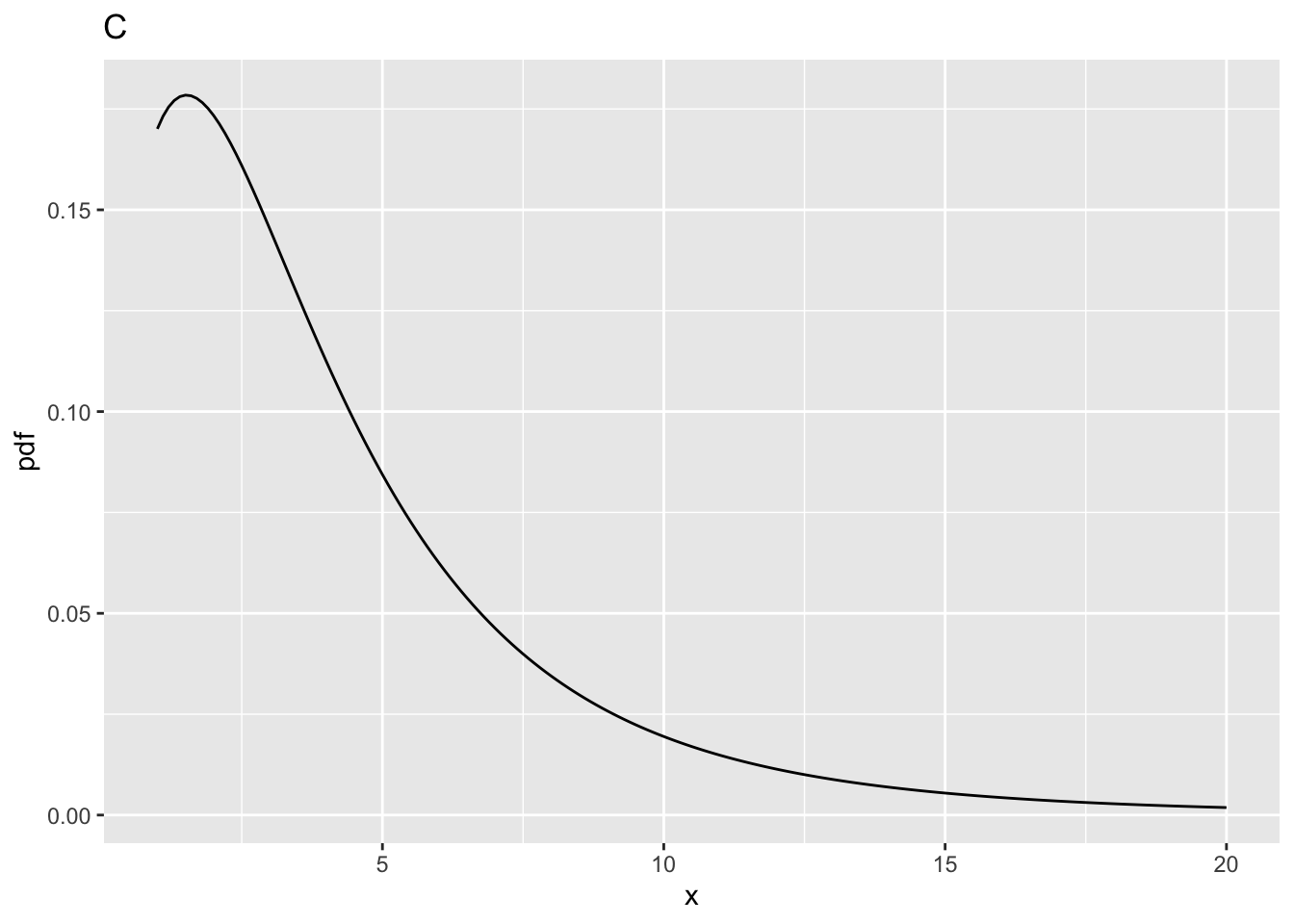
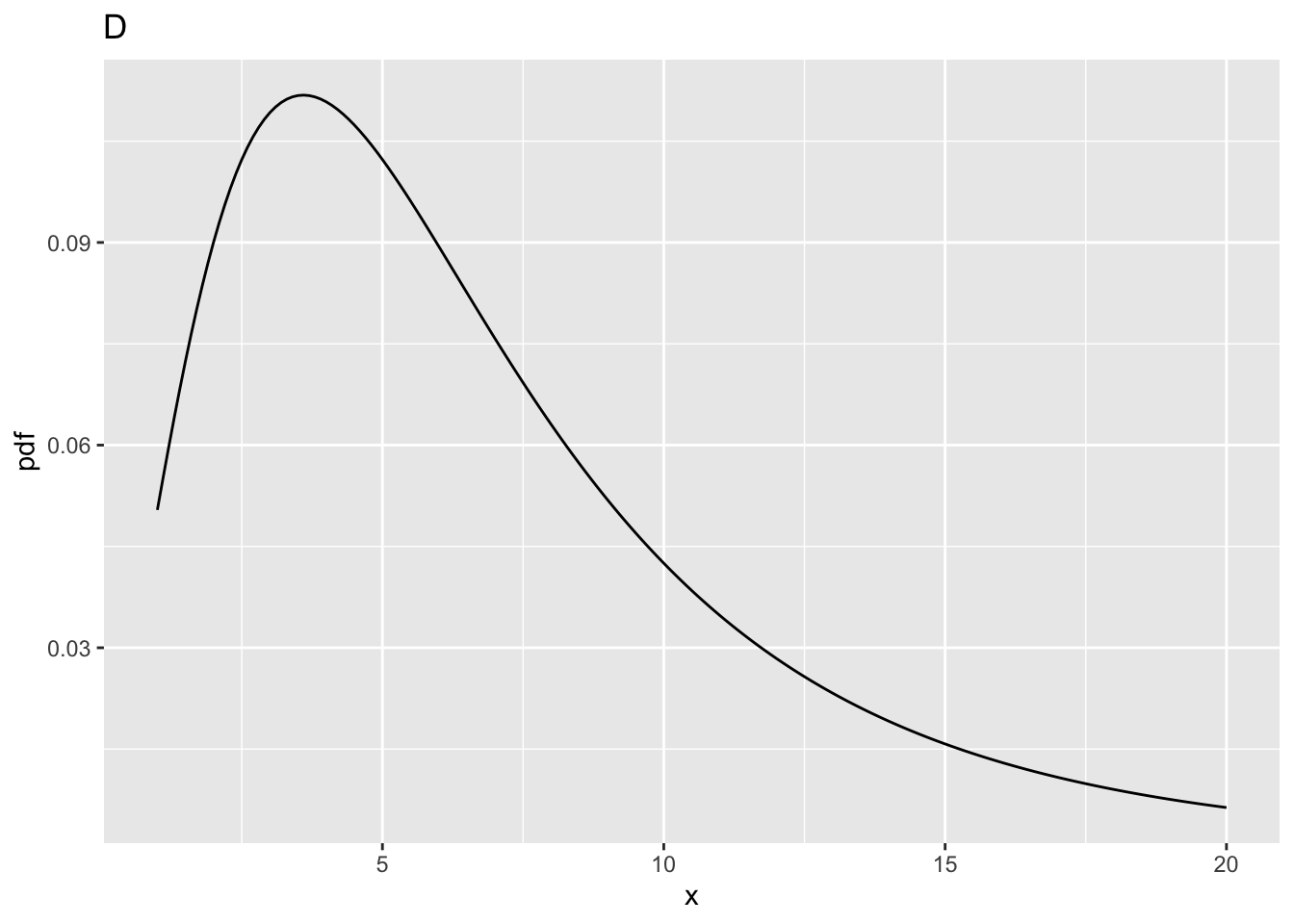
|
ndf
|
ddf
|
fCrit
|
ncp
|
pFgtFCrit
|
A
|
2
|
10
|
4.102821
|
0
|
0.0500000
|
B
|
2
|
10
|
4.102821
|
2
|
0.1775840
|
C
|
2
|
10
|
4.102821
|
5
|
0.3876841
|
D
|
2
|
10
|
4.102821
|
10
|
0.6769776
|
Summary
- Power increases with increasing
ddf
and ncp
.
- The effect of increasing
ncp
is quite dramatic. This is because power depends on the square of ncp
.
- As
ndf
increases, fCrit
decreases, which makes it more likely that the NH will be rejected.
- With increasing numbers of treatments the probability is greater that the F-statistic will be large enough to exceed the critical value.
Chakraborty, Dev P. 2017. Observer Performance Methods for Diagnostic Imaging: Foundations, Modeling, and Applications with r-Based Examples. Boca Raton, FL: CRC Press.
10.5 Comments
10.5.1 Fig. A
ncp = 0
, i.e., the central F-distribution.fCrit
in the above code block, is the value ofx
such that the probability of exceedingx
is \(\alpha\). The corresponding parameteralpha
is defined above as 0.05.fCrit
= 4.102821. Notice the use of the quantile functionqf()
to determine this value, and the default value ofncp
, namely zero, is used; specifically, one does not pass a 4th argument toqf()
.fCrit
. As expected,prob > fCrit
= 0.05 because this is howfCrit
was defined.10.5.2 Fig. B
ncp = 2
,ndf
= 2 andddf
= 10.prob > fCrit
= 0.177584, i.e., the statistical power (compare this to Fig. A whereprob > fCrit
was 0.05).10.5.3 Fig. C
ncp = 5
,ndf
= 2 andddf
= 10.prob > fCrit
= 0.3876841.10.5.4 Fig. D
ncp = 10
,ndf
= 2 andddf
= 10.prob > fCrit
is 0.6769776.x
= 4.102821, fraction 0.6769776 of the probability distribution in Fig. D lies to the right of this line10.5.5 Summary
The larger that non-centrality parameter, the greater the shift to the right of the F-distribution, and the greater the statistical power.